- Have a clear understanding of the different challenges and tasks machine learning can effectively solve.
- Understand the key steps to design, train and validate a machine learning model.
- Have a deep understanding of (generalised) linear models, which gives you good foundations for advanced ML topics.
- Have gained a practical understanding of what building a machine learning model in Python requires.
Are you ready to start your machine learning (ML) journey? In this course, I will introduce you to this revolutionary field. With a focus on "math light" explainers, hands-on exercises and real-world examples, our self-paced approach will give you a solid foundation in the core concepts of modern ML.
We will focus on insights and practical tips so that you can apply what you learn immediately in your own projects.
In this course we will cover:
- An overview of what machine learning is (and what it isn't), and the different types of tasks machine learning can effectively solve.
- The Machine Learning workflow: from preparing data and training your model to deploying and validating it in the wild.
- A deep dive into the linear models that give you the core foundations needed for building more advanced models.
- Detailed demos of 8 machine learning algorithms for you to try in your own projects.
We‘ll work on the basis that you’re pretty new to Python, but have some basic understanding of fundamental programming concepts and can run code locally on your machine.
If you feel like you could do with an introduction or a refresher, then take a look at Seán's free course which will get you up to speed on Python, Getting Started and Python Basics.
Section 1: Starting Blocks : Introduction to Machine Learning
In this first section, we introduce the world of machine learning and consider:
- what are the core concepts in machine learning?
- what are machine learning algorithms trying to do?
We will work through some high profile examples and talk about what's going on under the hood. We will focus on understanding the different types of task machine learning can accomplish, and then introduce you to the first two ML algorithms!
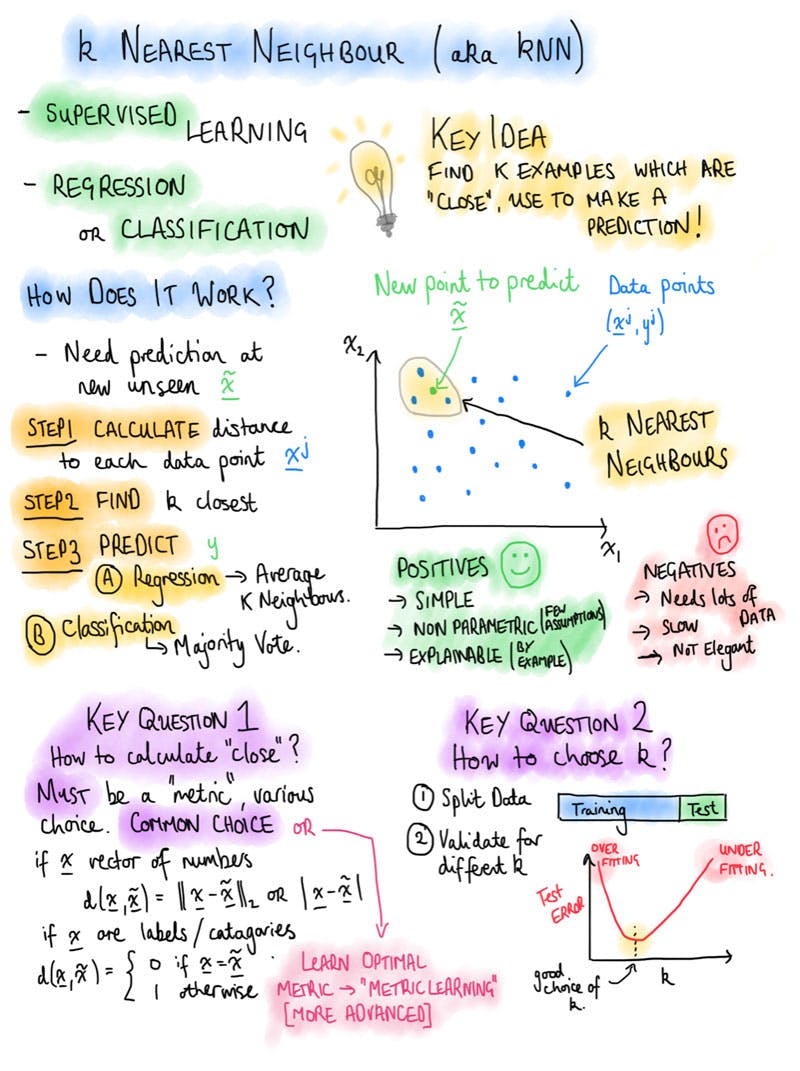
Fig 1. A mind-map of k-Nearest Neighbour - one of our first models.
Section 2: We're Off : Understanding the Machine Learning Workflow
Most machine learning projects have a natural flow. We want to give you a structure so that when you we start building machine learning models, the steps we take become familiar and second nature.
So, here we will walk you, step-by-step through the early data preparation and feature selection stages. We will then talk about model choice, training and tuning. Finally, we will focus on model validation and, ultimately, how you might deploy these models.
Section 3: Ninja Status at Linear Models
Ok, linear models might not seem that exciting. People think at first that they're just straight lines . . . . this is so wrong, and I love them! They provide the basics you need to understand advanced topics like deep neural networks or Gaussian Processes. To be honest, they underpin everything in ML.
In this final section, we will work through the key concepts of fitting both Linear and Generalised Linear Models (GLMs). We will talk about feature selection - which includes Predictive Power Score (or PPS) and Principle Component Analysis (PCA). We'll also cover the important topic of over-fitting and how we address this using regularisation methods.