Welcome to the fifth and final section of our course 'Machine Thinking' in which we shall introduce our last professional ML tool: Gradient Boosted Trees. A close relative of the Random Forest, boosting gradients zooms in on the convergence of an ensemble, using less complicated weak leaners--trees stripped down right to the tree stump. What does this mean? A light-weight, fast and effective algorithm.
We take a deep dive into a popular API for this technique: XGBoost, standing for 'eXtreme Gradient Boosting'. Possibly the most common deployment of a machine learning algorithm known for its predictive ability, high functionality and fine tuning.
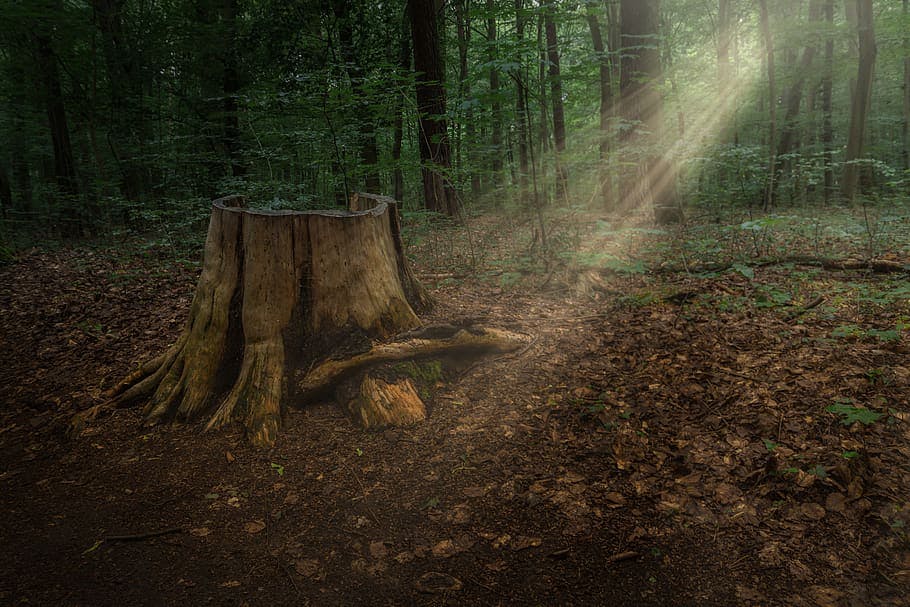
In this section we explore gradient boosting and XGBoost in the following:
- Introduction to the technique of Gradient Boosted Trees.
- Key mathematical construction for intuition.
- Worked data example: coding the algorithm from scratch.
- XGBoost in the wild: how does it fair on our prototype data.
- XGBoost functionality and cross validation.